De-identification, Anonymization, and Pseudonymization: What’s the Difference?
- Claude Annoh
- 2 days ago
- 3 min read
If you’ve ever worked on a research project involving interviews, patient data, or personal information, you’ve probably heard terms like anonymization, pseudonymization, and de-identification thrown around. But what do they actually mean in plain language? And how do you know which one you need? Let’s walk through what each term really means so you know how to protect your participants and stay on the right side of ethics and compliance.
Why This Even Matters
Say you just finished recording 10 interviews for your thesis or clinical research project. These are full of names, job titles, cities, and sensitive info your participants trusted you with. Now, you need transcripts. But before you hit "send" to your transcription service, you wonder, "Am I protecting this data enough?" That's where these three terms come in: de-identification, anonymization, and pseudonymization.
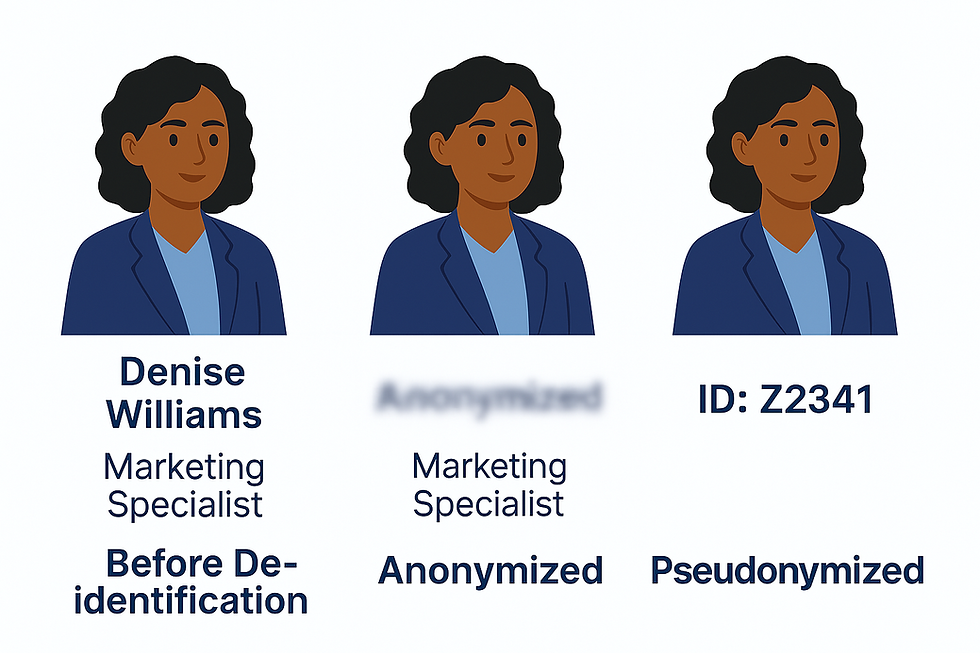
1. What is De-identification?
De-identification is basically a way of saying "Let’s remove anything that points back to who this person is." It’s the general process of scrubbing a transcript or dataset of personal identifiers, like someone’s full name, phone number, or where they work.
Example:
Original: “My name is Sarah Williams, I work at Mount Sinai in New York.”
De-identified: “My name is [Participant], I work at a hospital in the New York.”
You’re not erasing their voice, but you’re just making sure no one reading or hearing the data can say, “Oh, I know who that is.”
2. What is Anonymization?
Anonymization goes a step further than de-identification. It means there’s no way to trace the data back to the person at all. Not even you, the researcher. You remove all identifiers, and there’s no key, code, or spreadsheet somewhere that could reverse the process.
Example:
You interview 30 patients for a healthcare study. You remove their names, their cities, the name of the hospital, even specific job roles. You label them "Participant 1," "Participant 2," and so on. The data is now fully anonymous. No one, not even you, could identify them again.
This is usually what you want when you're:
Submitting research to a journal
Sharing data publicly
Finishing a study where follow-up isn’t needed
3. What is Pseudonymization?
Now here’s where it gets a little more practical. Pseudonymization is when you replace identifying details with fake names or codes, but you still keep a separate file that links those codes back to the real person. It’s super useful when you want to protect people’s identities but still need to track their data later on.
Example:
In a two-year study, you interview “John Smith” and label his transcript as “Participant 102.” You keep a secure spreadsheet linking “102” to “John Smith,” but you never share that file with anyone else.
You can still reach back out to John later if you need follow-up, but to everyone else, he’s just Participant 102.
This approach is common in:
Clinical trials
Longitudinal research
Studies where follow-up interviews or check-ins are needed

Comparison Chart
Term | Can You Trace It Back? | Common Use Case |
De-identification | Sometimes | Internal file-sharing, early research stages |
Anonymization | No | Journals, public sharing, archiving |
Pseudonymization | Yes (with a key) | Long-term studies, clinical research |
So Which One Should You Use?
It depends on what you're doing:
If you’re just sharing files with your supervisor or co-researcher? De-identify.
If you’re publishing or sharing with the public? Anonymize.
If you need to follow up with participants later? Pseudonymize.
When in doubt, go with the safest option and talk to your IRB or advisor if you’re not sure.
How We Help at Qualtranscribe
At Qualtranscribe, we help researchers all over the world protect their participants while getting clean, accurate transcripts. Whether you need full anonymization, coded pseudonyms, or just some basic de-identification, our human transcription team can tailor the transcript to your project.
We’ve worked with:
University researchers preparing data for IRBs
Healthcare teams following HIPAA rules
Social scientists working with multilingual focus groups
PhD students who just want to get it done right
Your research deserves protection. So do your participants. Knowing how to handle sensitive data properly isn’t just a compliance issue, it’s about trust. And that trust starts with how you manage the words people share with you.